In the ever-evolving field of technology, nn models have emerged as a pivotal component that drives innovation and efficiency. These models, known for their ability to simulate human-like decision-making processes, have transformed industries ranging from healthcare to finance. As we delve deeper into the intricacies of nn models, we will uncover their significance, functionality, and the future they promise to shape.
Understanding nn models requires a blend of technical knowledge and practical application. They utilize complex algorithms to process vast amounts of data, identify patterns, and make predictions that can significantly enhance operational efficiency. This capability is particularly valuable in sectors where data-driven decisions are crucial, such as marketing, customer service, and product development.
As we navigate through this article, we will address common questions and concerns about nn models, shedding light on their mechanics and potential. From their underlying principles to their real-world applications, our exploration will provide a comprehensive understanding of this fascinating subject.
What Are nn Models and How Do They Work?
nn models, or neural network models, are computational frameworks inspired by the human brain's architecture. These models consist of interconnected nodes, or neurons, that process information through layers. The primary function of nn models is to recognize patterns and make predictions based on input data. Here’s how they work:
- Input Layer: This layer receives the raw data.
- Hidden Layers: These layers perform computations and extract features from the data.
- Output Layer: This layer produces the final prediction or classification.
What Are the Key Components of nn Models?
Understanding the core components of nn models is essential for grasping their functionality. The primary elements include:
- Neurons: The basic units that process information.
- Weights: Parameters that adjust as the model learns from data.
- Activation Functions: Functions that determine the output of a neuron.
- Learning Rate: A hyperparameter that controls how much the model adjusts weights during training.
What Are the Applications of nn Models?
nn models have a wide array of applications across various fields. Some of the most notable include:
- Healthcare: Predicting patient outcomes and diagnosing diseases.
- Finance: Fraud detection and risk assessment.
- Marketing: Customer segmentation and targeted advertising.
- Autonomous Vehicles: Driving behavior prediction and navigation.
Who Are the Pioneers Behind nn Models?
To appreciate nn models fully, it is essential to recognize the key figures who have contributed to their development. Among these are:
Name | Field of Expertise | Major Contribution |
---|---|---|
Geoffrey Hinton | Computer Science | Developed backpropagation algorithm. |
Yann LeCun | Artificial Intelligence | Pioneered convolutional neural networks. |
Yoshua Bengio | Machine Learning | Contributed to deep learning techniques. |
How Do nn Models Learn from Data?
The learning process of nn models involves training on large datasets. During training, the model adjusts its weights based on the errors it makes in predictions. This process is known as backpropagation, which helps minimize the difference between the predicted output and the actual output. The steps in this learning process are:
- Feedforward: Input data is passed through the network to generate an output.
- Loss Calculation: The difference between the predicted output and actual output is calculated.
- Backpropagation: The model adjusts weights to minimize loss.
- Iteration: This process is repeated for multiple epochs until the model converges.
What Are the Challenges Associated with nn Models?
Despite their advantages, nn models face several challenges that can impact their performance. These include:
- Overfitting: When a model learns the training data too well, it may fail to generalize to new data.
- Data Requirements: nn models typically require large amounts of data to train effectively.
- Computational Costs: Training complex nn models can be resource-intensive.
- Interpretability: Understanding how a model arrives at its decisions can be difficult.
What Is the Future of nn Models?
The future of nn models looks promising, with advancements in technology and research paving the way for more sophisticated and efficient models. Researchers are focusing on:
- Improving Efficiency: Developing methods to reduce computational costs.
- Enhancing Interpretability: Creating models that are easier to understand.
- Expanding Applications: Exploring new domains for nn model applications.
- Ethics and Fairness: Addressing biases in data to ensure fairness in model predictions.
How Can One Get Started with nn Models?
For those interested in diving into the world of nn models, several resources and steps can help kickstart your journey:
- Learn the Basics: Familiarize yourself with fundamental concepts in machine learning and neural networks.
- Online Courses: Enroll in courses that provide hands-on experience with nn models.
- Join Communities: Engage with online forums and communities to share knowledge and experiences.
- Experiment: Start building simple nn models using frameworks like TensorFlow or PyTorch.
In conclusion, nn models represent a transformative technology with vast potential across various sectors. Their ability to mimic human decision-making processes while processing vast amounts of data makes them invaluable tools in today's data-centric world. As we continue to explore their applications, it is clear that the journey of nn models is just beginning, and the possibilities are truly limitless.


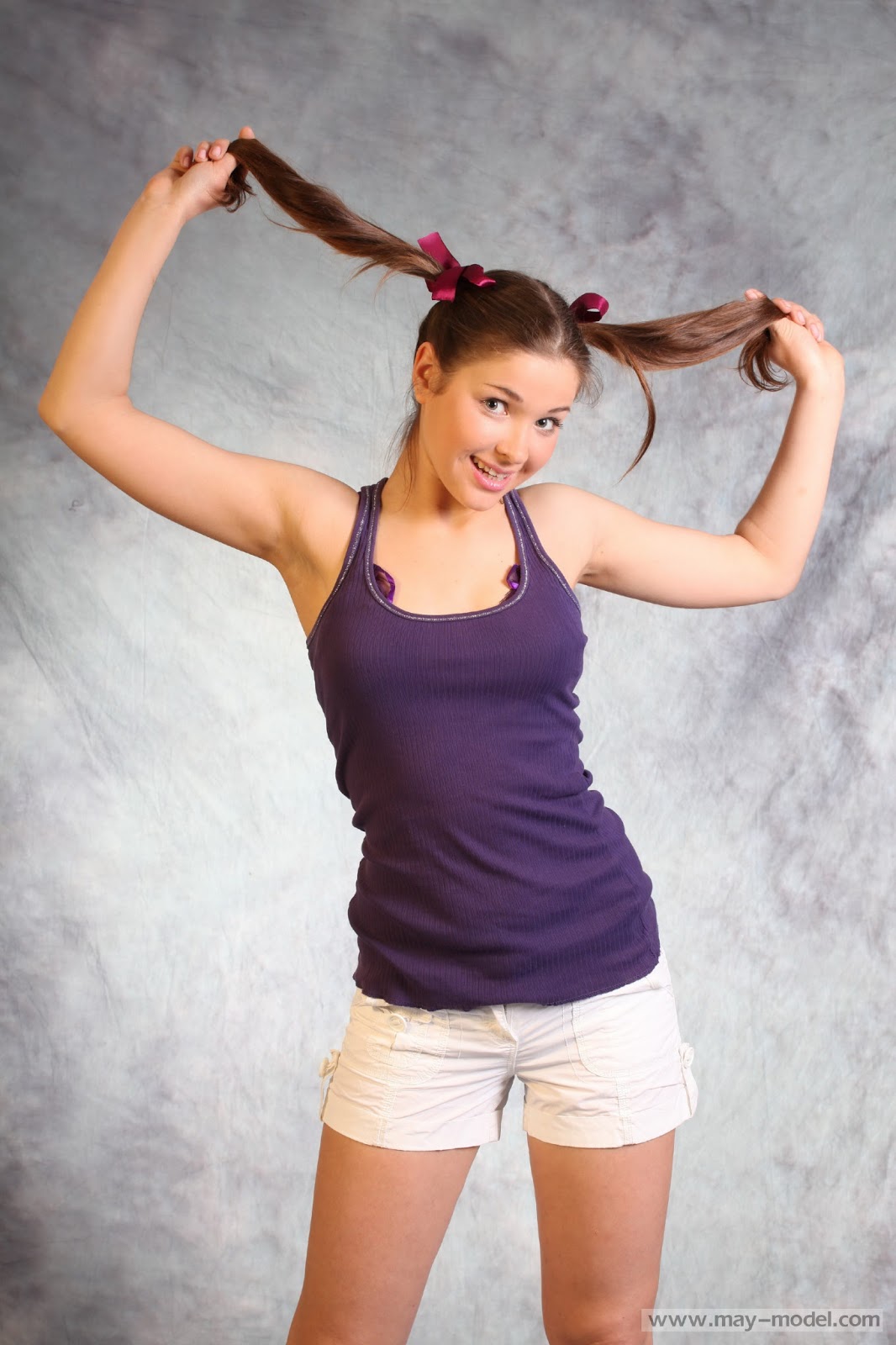.jpg)